Matthew Bennett received a master’s in Industrial and Organizational Psychology from the University at Albany in 2022 and works as a research scientist and psychometrician at SHL Global, one of the largest providers of talent acquisition and management services to clients worldwide. He is currently pursuing advanced certification in machine learning and artificial intelligence through the University at California, Berkely. His current research interests include Thurstonian IRT in assessment, the use of machine learning and artificial intelligence in psychometric applications, and the crossroads of neurodivergence and psychometric assessment. In his free time, he enjoys hiking the nature trails of Upstate New York.
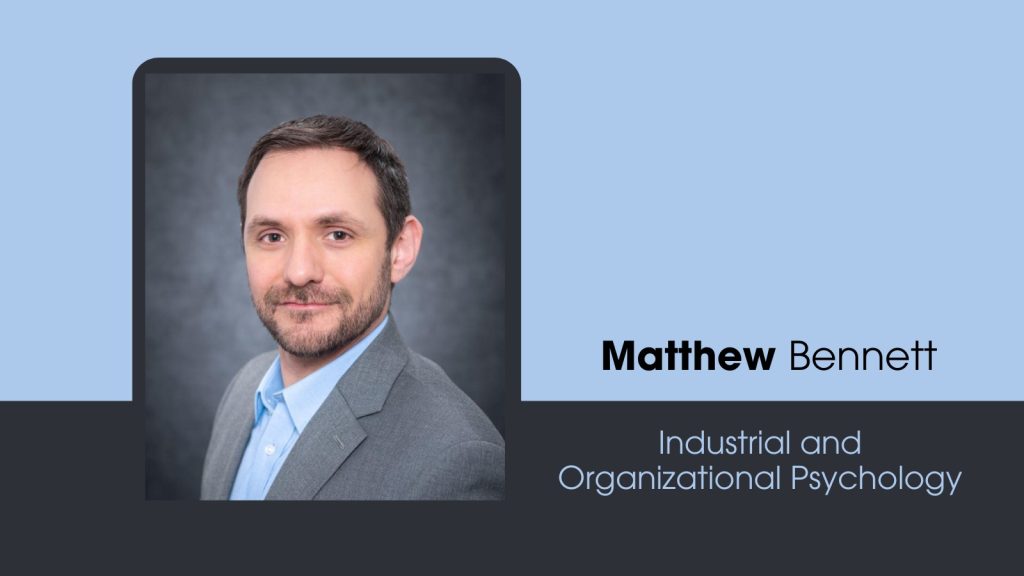
The Social Digest: Can you share examples of key performance metrics or indicators that organisations can use to measure employee productivity effectively?
The KPIs that best indicate performance are probably going to change a bit from organisation to organisation. In general, I think the best metrics come in the form of manager ratings. There are a lot of factors that can affect objective metrics (like amount of sales in a month) that can make it difficult to compare employee performance across business locations or across time. Plus, these numbers often miss out on bigger picture facets of performance, such as how proactive an employee is or how well they collaborate across teams, and objective metrics can’t account for temporary situations that may negatively (or positively) affect an employee’s productivity. An otherwise excellent employee shouldn’t be flagged as unproductive because their partner is going through a cancer scare and they underperformed last quarter. A good manager’s insight into employee performance is one of the most valuable metrics you can have, and structured ratings forms that ask questions about specific performance areas are going to give the best ratings. Any objective metrics used to measure performance should be used to supplement to these kinds of human judgment ratings.
The Social Digest: How can data-driven insights help organisations identify and address potential barriers or challenges to employee productivity?
That’s a great question. As a psychometrician in the talent acquisition space, I advocate for the use of objective assessment data to uncover crucial insights into employee capability, workplace personality, and potential. Good assessment data, used effectively, has a high ROI because it can identify strengths and growth areas in individuals and in teams, which can be used in a variety of ways to maximize workflows within an organization. For example, do you have a lot of employees with great technical skills in one department, and a lack of these experts in another department? Reorganization or creating cross-functional collaborations may eliminate siloes and alleviate the barriers that individual teams face. Additionally, other techniques like organizational network analysis can provide insights about how connected individuals and teams are. Productivity issues are often the result of poor communication networks, when vital knowledge and resources aren’t effectively shared across (or within) teams.
The Social Digest: What methods or tools do you recommend for collecting and analysing employee data while ensuring privacy and ethical considerations?
Another great question! I would bring the conversation back to objective assessment data and managerial ratings. It’s easier to avoid ethical dilemmas with these measures. It’s become common practice to scrape information off social media or harvest KPI data from personnel management software to infer employee information, but these are fraught with ethical dilemmas and often yield questionable results. You’re trying to get information about someone through secondary sources, which distorts the information. Additionally, observing employee activity through methods like keyloggers or monitoring presence on an organizational communications software (like Microsoft Teams) can be invasive, and collecting these kinds of data suggest that an organization cares more about employees looking busy rather than employees performing well.
There are other factors related to consent and transparency, too. Employees should be made aware of what you’re doing, and you should actively seek both their consent and their feedback. If employees find out you’re doing things behind their back or have buried a notification of your data collection twenty paragraphs into the employee handbook, they may not all quit immediately—but they’re not going to be as loyal or as productive.
The Social Digest: What strategies do you recommend for fostering a culture of data-driven decision-making within an organisation, particularly among frontline managers and supervisors?
Developing this kind of culture sometimes requires a significant shift away from standard operating procedure. People tend to make decisions with their gut, rather than empirical data. It’s critical to get buy-in from managers and supervisors because they tend to work most closely with the employees who will be doing the bulk of the work in many organizations. If they don’t see the value in investing the effort to gather and analyse quality data, both the data collection and decision-making processes will suffer, and may even be rendered ineffectual.
To facilitate this kind of shift, ensure that managers and supervisors receive the training and support necessary to engage effectively in the process. Additionally, it’s important to actively listen to and address their concerns or reservations and incorporate their feedback. Very often, procedures for gathering and using employee performance data may look good from the perspective of the technical experts crafting the procedures and the executive signing off on them, but they may have to be adjusted to fit into the work environment effectively.
The Social Digest: How can industrial psychology principles be integrated into talent management practices to optimise employee engagement and performance?
I think the most important insight from IO psychology is that people are people. When people are treated like numbers on an accounting sheet, you create a toxic environment that is detrimental to organizational goals. Research consistently shows that employees are more engaged and loyal when they perceive organizational policies, including compensation, as fair. This not only enhances the quality of their work and makes them stay at companies longer, but it also maximizes their contributions to the short- and long-term success of their organization.
The world is changing rapidly, and it’s important to take a big-picture approach. Most of the workforce is now disillusioned with popular business models that evaluate everything in terms of the next quarter’s financial report. Ennui and dissatisfaction with the system are a root cause for many of the recent social movements, from the growth of union activity in America to bai lan in China. Around the world, populations are declining, and it is becoming increasingly important for organizations to retain high-quality talent. To do that, employees need to feel like they’re treated fairly. IO psychology brings a lot of insights to the table, but most others pale in comparison to the above.
The Social Digest: Looking ahead, what trends or advancements do you foresee in the field of industrial psychology regarding the use of data analytics to enhance employee productivity?
IO psychology takes a measured approach to adopting new technologies because we prioritize evidence-based methods verified by the scientific method. This has the (significant) benefit of grounding our recommendations in what works, but it also means we are sometimes slow to embrace new innovations. I don’t think that’s going to change but, in the age of artificial intelligence (AI), the field must work hard to catch up. I see a lot of excitement and interest among IOs to do just that, particularly around the data and tech space.
Right now, the best thing that many organizations can do to improve employee productivity is to empower workers to incorporate large language models (LLMs) and similar AI agents into their workstreams. (Enable, don’t replace—a few average employees with these tools will be much more productive than one star employee.) Right now, for most organizations, this is going to have a higher ROI than using analytics to (re-)organize your workforce.
After that, I would say that adopting a talent intelligence approach—combining assessment data and performance management systems and using both to enhance internal mobility—is one of the best analytics-driven approaches that organizations can adopt for enhancing productivity.
The Social Digest: What safeguards or protocols do you recommend implementing to mitigate the risk of bias or discrimination when utilising data analytics in hiring, promotion, or performance evaluation processes?
Great question. I would caution against the use of “inferred” information about employee performance. That would include anything that is about a candidate or employee but that isn’t an active measurement and can include social media data and KPI metrics. This information usually gives a distorted view of a person and it is often biased against certain vulnerable groups. It’s better to get direct information through assessments that have undergone a rigorous creation and evaluation process.
Reputable vendors should have thorough technical documentation of an assessment’s psychometric reliability and validity (two key concepts that anyone purchasing such assessments should know about), and data showing that it isn’t unfairly biased against certain demographics. And all that goes double for new, AI-powered tools that use “black box” algorithms to assess candidates. These tools can be good, but there are many products on the market that would be damaging for organizations to use because there were no safeguards in their creation to ensure they’re reliable, valid, and fair.
Also, run regular audits on your hiring and promotion pipelines to ensure that there isn’t discrimination. Even with all these safeguards, it’s possible that internal stakeholders are misusing tools, which can cause bias.
The Social Digest: In the context of high-stakes decision-making, such as in healthcare or public safety, how can organisations balance the benefits of data-driven insights with ethical considerations and human judgement?
I’d emphasize the vital role of dedicated teams tasked with overseeing data analytics processes. There’s a lot that goes into ensuring ethical use of data in modern organizations, including reviewing data pipelines and algorithms used, examining how data and analytics impact business decisions that affect customers and the public, and being aware of and managing externalities to the use of these tools. Organizations must thoroughly review their data pipelines and algorithms to guard against biases that might affect decision-making. For instance, datasets used to train machine learning models or create dashboards can unintentionally discriminate against specific demographics, due to uneven representation. Another concern is that, without proper oversight, using analytics to shape employee behaviour may result in perverse incentives where employees focus on meeting some arbitrary metric of busy-ness, rather than productivity, which both unduly burdens employees and gets in the way of providing effective services.
It’s imperative to have a watchdog mechanism. This oversight group should not only monitor compliance with ethical standards but also have a real impact on business decisions to prevent unethical practices, even if they might seem beneficial in the short term. They will be vital to long-term organizational health and effectiveness.
This interview was conducted by The Social Digest on 05/05/2024. If you have any interview recommendations or have a story that you want to share with our readers, write us at editor@thesocialdigest.com